
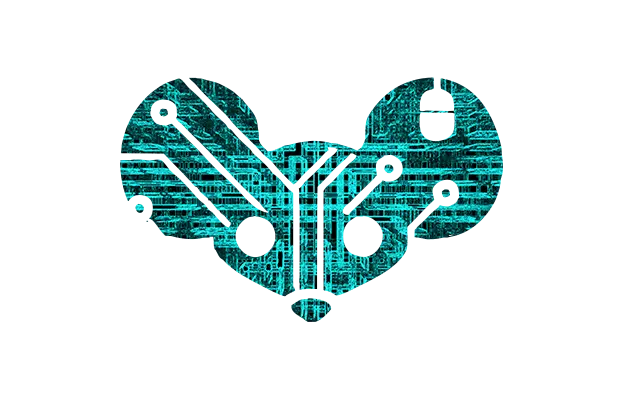
Yeah, you can certainly get it to reproduce some pieces (or fragments) of work exactly but definitely not everything. Even a frontier LLM’s weights are far too small to fully memorize most of their training data.
Yeah, you can certainly get it to reproduce some pieces (or fragments) of work exactly but definitely not everything. Even a frontier LLM’s weights are far too small to fully memorize most of their training data.
Most “50 MP” cameras are actually quad Bayer sensors (effectively worse resolution) and are usually binned 2x to approx 12 MP.
The lens on your phone likely isn’t sharp enough to capture 50 MP of detail on a small sensor anyway, so the megapixel number ends up being more of a gimmick than anything.
I agree with your thoughts. I hate what Bambu has done to the industry in terms of starting a patents arms race and encouraging other companies to reject open source, but I do love how they’ve pushed innovation and have made 3D printing easier for people just looking for a tool.
I hope the DIY printers like Voron, Ratrig, VzBot, and E3NG can continue the spirit of the RepRap movement.
I work in an area adjacent to autonomous vehicles, and the primary reason has to do with data availability and stability of terrain. In the woods you’re naturally going to have worse coverage of typical behaviors just because the set of observations is much wider (“anomalies” are more common). The terrain being less maintained also makes planning and perception much more critical. So in some sense, cities are ideal.
Some companies are specifically targeting offs road AVs, but as you can guess the primary use cases are going to be military.
Some apps only require ‘basic’ play integrity verification, but now check to see if they’re installed via the Play Store. They refuse to run if they’re installed via an alternative source.
This has been a problem for GrapheneOS, since some apps filter themselves out of the Play Store search if you don’t pass strong play integrity, despite the fact that they don’t require it. Luckily Graphene now had a bypass for this.
OBS can use NVENC, though IIRC it needs to be built with support enabled, which may not be the case for all distros’ package managers.
Yep, since this is using Gaussian Splatting you’ll need multiple camera views and an initial point cloud. You get both for free from video via COLMAP.
Yeah, in typical Google fashion they used to have two deep learning teams: Google Brain and DeepMind. Google Brain was Google’s in-house team, responsible for inventing the transformer. DeepMind focused more on RL agents than Google Brain, hence discoveries like AlphaZero and AlphaFold.
The general framework for evolutionary methods/genetic algorithms is indeed old but it’s extremely broad. What matters is how you actually mutate the algorithm being run given feedback. In this case, they’re using the same framework as genetic algorithms (iteratively building up solutions by repeatedly modifying an existing attempt after receiving feedback) but they use an LLM for two things:
Overall better sampling (the LLM has better heuristics for figuring out what to fix compared to handwritten techniques), meaning higher efficiency at finding a working solution.
“Open set” mutations: you don’t need to pre-define what changes can be made to the solution. The LLM can generate arbitrary mutations instead. In particular, AlphaEvolve can modify entire codebases as mutations, whereas prior work only modified single functions.
The “Related Work” (section 5) section of their whitepaper is probably what you’re looking for, see here.
40 series to 30 series was pretty tangible IMO (4090 gets something like 30-50% more perf in most tasks than 3090 Ti with the same TDP), in part thanks to the much higher L2 cache plus newer process node.
50 series was very poor though, probably because it’s the same process node.
No they are not “a tool like any other”. I do not understand how you could see going from drawing on a piece of paper to drawing much the same way on a screen as equivalent as to an auto complete function operated by typing words on one or two prompt boxes and adjusting a bunch of knobs.
I don’t do this personally but I know of wildlife photographers who use AI to basically help visualize what type of photo they’re trying to take (so effectively using it to help with planning) and then go out and try and capture that photo. It’s very much a tool in that case.
Unfortunately proprietary professional software suites are still usually better than their FOSS counterparts. For instance Altium Designer vs KiCAD for ECAD, and Solidworks vs FreeCAD. That’s not to say the open source tools are bad. I use them myself all the time. But the proprietary tools usually are more robust (for instance, it is fairly easy to break models in FreeCAD if you aren’t careful) and have better workflows for creating really complex designs.
I’ll also add that Lightroom is still better than Darktable and RawTherapee for me. Both of the open source options are still good, but Lightroom has better denoising in my experience. It also is better at supporting new cameras and lenses compared to the open source options.
With time I’m sure the open source solutions will improve and catch up to the proprietary ones. KiCAD and FreeCAD are already good enough for my needs, but that may not have been true if I were working on very complex projects.
Cute cat! Nevermore and Bentobox are two super popular ones.
Since you’re running an E3 V2, first make sure you’ve replaced the hotend with an all-metal design. The stock hotend has the PTFE tube routed all the way into the hotend, which is fine for low temp materials like PLA, but can result in off-gassing at higher temperatures such as those used by ASA and some variants of PETG. The PTFE particles are almost certainly not good to breathe in during the long term, and can even be deadly to certain animals such as birds at small quantities.
In my experience doing a bit more than 10% can be helpful in the event of underextrusion, plus I’ve seen it add a bit more rigidity. But you’re right that there are diminishing returns till you start maxing out the infill.
4 perimeters at 0.6mm or 6 at 0.4 should be fine.
Yeah, I agree. In the photo I didn’t see an enclosure so I said PETG is fine for this application. With an enclosure you’d really want to use ABS/ASA, though PETG could work in a pinch.
I also agree that an enclosure (combined with a filter) is a good idea. I think people tend to undersell the potential dangers from 3D printing, especially for people with animals in the home.
Thanks for the respectful discussion! I work in ML (not LLMs, but computer vision), so of course I’m biased. But I think it’s understandable to dislike ML/AI stuff considering that there are unfortunately many unsavory practices taking place (potential copyright infringement, very high power consumption, etc.).
All good, it’s still something to keep in mind (especially if OP thinks about enclosing their printer in the future). Thanks for your comment!
IMO heat formed from stress will not be a big deal, especially considering that people frequently build machines out of PETG (Prusa’s i3 variants, custom CoreXYs like Vorons and E3NG). The bigger problem is creep, which suggests that you shouldn’t use PLA for this part.
PETG will almost certainly be fine. Just use lots of walls (6 walls, maybe 30% infill). PETG’s heat resistance is more than good enough for a non-enclosed printer. Prusa has used PETG for their printer parts for a very long time without issues.
Heat isn’t the issue to worry about IMO. The bigger issue is creep/cold flowing, which is permanent deformation that results even from relatively light, sustained loads. PLA has very poor creep resistance unless annealed, but PETG is a quite a bit better. ABS/ASA would be even better but they’re much more of a headache to print.
For real though, Gojo soap seems to work the best for getting rid of grease and oil from machines. My guess is regular soaps don’t do a great job at carrying away the oil residue, but Gojo soap just sands down your top skin layer to remove it.