
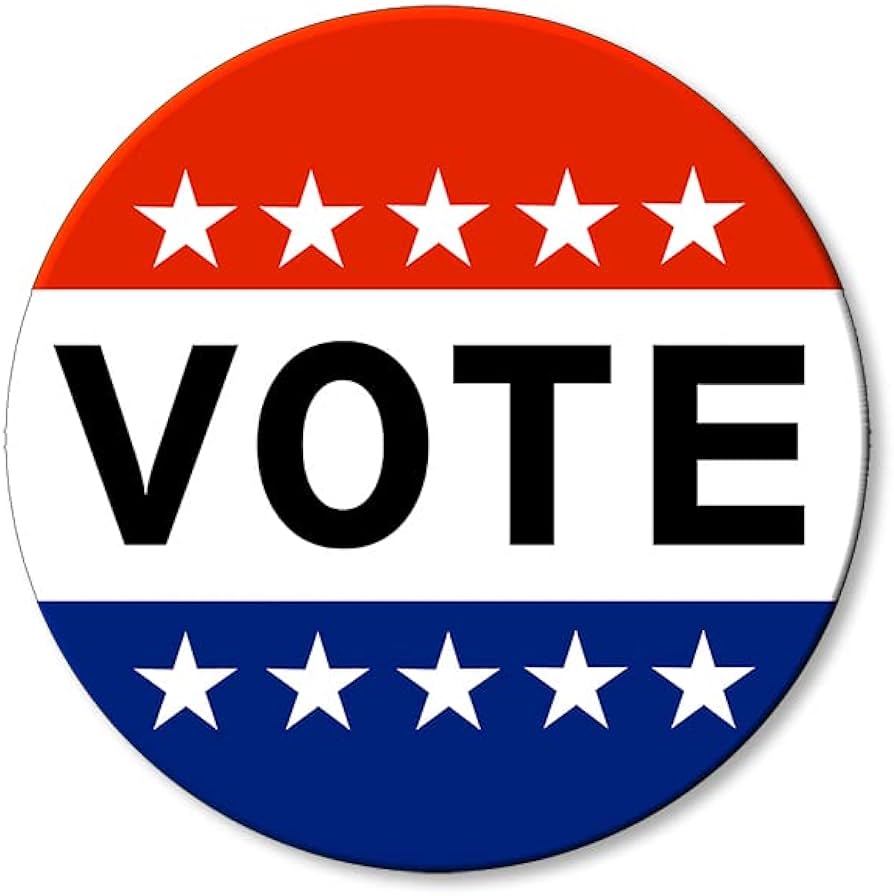
No source but I remember musk saying that the tariff change would require a rough transition that Americans would need to go through to get to good times or whatever.
I though that that was to prepare their base to the actual shitstorm and to them promise them the good times or whatever.
Is this PR comment forever gone now that they are in power?
I would glare you back and explain for the 98th time that in my field software being okay doesn’t mean that it will run. Data quality and config files that are not out purview can make correct software crash.
It’s not data engineering in this case though so I mostly agree with you.